Data management: what is it, why is it more important than ever, how is it evolving, and what is the role of data management in creating value?
Data Management is the development, execution, and supervision of plans, policies, programs, and practices that deliver, control, protect, and enhance the value of data and information assets throughout their lifecycles.
Data has always made the online and digital world go round. As digital technologies and data play an increasingly important role in all facets of our lives, one could say that data today makes the world as we know it go round, including our physical environment as borders between ‘digital’ and ‘physical’ blur.
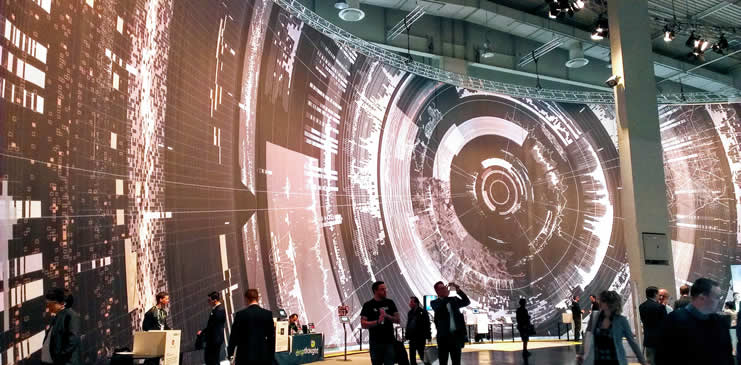
Seeing and understanding the incredible role that data plays pretty much everywhere, often even without us realizing it, is probably the best way to grasp the importance of data management. If data is vital and value, drives digital transformation, and is behind so much of what we do each day, ‘managing’ it must be equally crucial.
And managing data is indeed what data management is all about: making sure it’s properly used and delivers the right value and insights at the right time for the right reasons to the right people.
The other way around this, among others, means that avoiding the quality of data is bad, ensuring that the way it’s used is ethical and that it doesn’t fall into the wrong hands are some areas data management deals with.
Managing data means many things as data is everywhere, comes from numerous sources and can be leveraged in multiple ways before becoming actionable, usable, or unnecessary. Even if data is not needed anymore, we’re still managing it by deciding what must be done with it.
Data management – a holistic and collaborative approach
In other words: data management covers pretty much the entire lifecycle of the data we have/acquire/use/need.
The discipline of data management encapsulates many data management areas: from data governance and data architecture to data quality, data storage, and data protection/security. In that sense, you can compare data management with information management.
As you can see in the older graphics on information management in our information management guide, ensuring quality, security, storage, delivery, and business value of information is essential in this domain as well. If you already wonder what the difference between data and information and between data management and information management is, we cover that briefly later.
So, data management is a multifaceted but also multidisciplinary field. In recent years, i.a. due to data management challenges and evolutions requiring new methods, there has been a growing awareness that collaboration is essential.
A holistic view of data management and how to extract value from data requires collaboration between data experts across multiple data-related disciplines. However, a collaboration between the ‘data consumers,’ data creators, and data experts is inevitable in this data age too.
Moreover, data creators and data consumers are increasingly non-human in a more ‘connected’ era where the Internet of Things represents the fastest-growing data creation segment. Intelligent devices and machines, for instance, also need to consume data as we continue to automate, among others, in Industry 4.0.
An evolving field with numerous drivers
In other words: data management is also an evolving field. The realization that better collaboration and better processes are needed, for instance, led to DataOps. This relatively new discipline will play a vital role in big data analytics, artificial intelligence, machine learning, and many modern applications.
Although data management exists for a long time, attention for the discipline has grown with the advent of big data, the increasing importance of analytics, and the evolution to a more data-driven digital economy in which data is a business asset.
The arrival of stringent personal data protection measures across the globe and the ongoing proliferation of data sources/types is equally essential here. And so is the need for data storage (from databases and data warehouses to data lakes and data centers), security, and quality in a world where data has been dubbed the new oil/gold. Last but not least (for now), data management has also become more critical and complex because our data age and what we do with very diverse types and volumes of data are more complex than ever.
We’ll cover some of the main drivers of the evolutions in data management, but no doubt you can think of many. On that list of drivers, to summarize a bit:
- the fact that data has become a key business asset,
- the ongoing digitization and digitalization,
- the phenomenon of digital transformation,
- the addition of ever more data sources and types of data (the Internet of Things, social media, you name it),
- the challenges to extract value from data as volumes and variety of data continue to grow,
- the essential role of data analytics and speed at which insights are often required,
- industrial automation and the advent of Industry 4.0,
- evolving views on data privacy, data protection, and data security,
- the requirements of technologies such as artificial intelligence and machine learning,
- the migrations towards cloud computing in the enterprise with cloud data management on the rise,
- the fact that thus far, very few companies manage to unlock the value of data and information, and that the majority of data management investments/projects fails to deliver whereby changes on various fronts are inevitable,
- and much more.
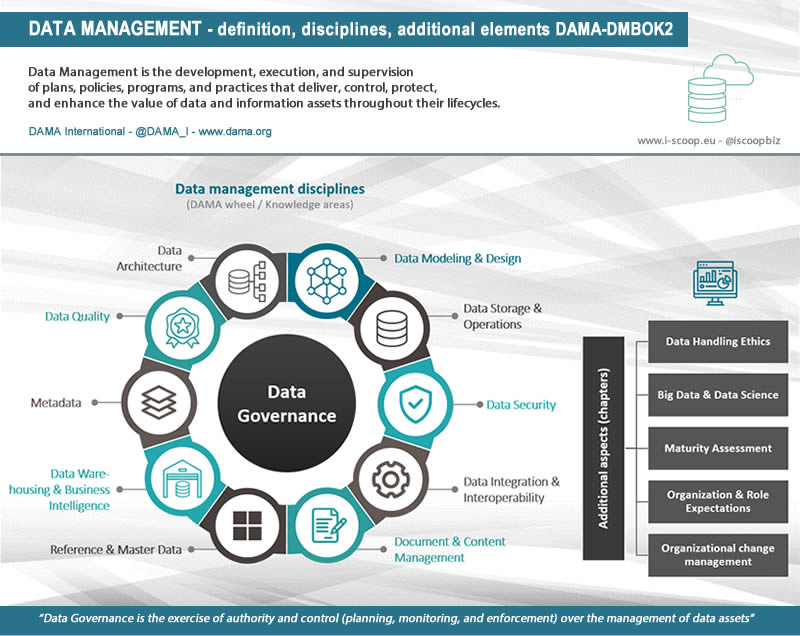
Data management definition and categorization of practices
Time for a definition of data management. DAMA International, an organization of data management professionals, defines data management as follows:
“Data Management is the development, execution, and supervision of plans, policies, programs, and practices that deliver, control, protect, and enhance the value of data and information assets throughout their lifecycles.”
The organization also categorized the broad range of data management activities in its “DAMA International’s Guide to the Data Management Body of Knowledge.”
They are known as the eleven Knowledge Areas of the DAMA-DMBOK Data Management Framework or DAMA Wheel by professionals and are:
- Data governance (at the center);
- Data architecture;
- Data modeling and design;
- Data storage and operations;
- Data security;
- Data integration and interoperability;
- Document and content management;
- Reference and master data;
- Data warehousing and business intelligence (BI);
- Metadata;
- Data quality.
“Data Governance is the exercise of authority and control (planning, monitoring, and enforcement) over the management of data assets” (DAMA)
Since edition 2, known as DAMA-DMBOK2, which you can buy from DAMA here, chapters have been added on topics that are very important these days:
- Data handling ethics;
- Big data and data science;
- Data management maturity assessment;
- Data management organization and role expectations;
- Data management and organizational change management.
These topics are important for previously mentioned reasons, such as the fact that data really is what digital business and the digital economy are very much about. But more on that later. We don’t cover all data management topics on our site as there’s a lot of (good) work but mainly cover data management evolutions (and new solutions for new challenges).
The difference between data and information management
As promised, a few words on the differences between data and information management.
It’s important to realize that papers, articles, and even books have been written on this topic (and on related domains such as content management and records management, and how they fit in this context) and that the views depend on whom you’re talking to.
The management of data and information for various purposes goes back to the early days of information technology. IT management, data management, and information management are closely related and intertwined.
Moreover, the various disciplines in each field are connected within their field as such and with domains in the other fields or even in areas that are not strictly about IT or data. An example: data security is related to, for instance, data storage. It is also related to cybersecurity, compliance and regulations (e.g. in life sciences, finance, and the context of personal data), risk management, etc.
Last but not least, we shouldn’t forget the domain of OT (Operational Technology) either. Industrial applications and systems create and use data since long before the so-called ‘Industrial IoT‘ exists. This data is also managed, even more so with the integration of IT and OT, leading to ‘smart industry‘, and the cyber-physical convergence of Industry 4.0.
Data management nowadays is mainly seen as a subset of information management in this complex landscape.
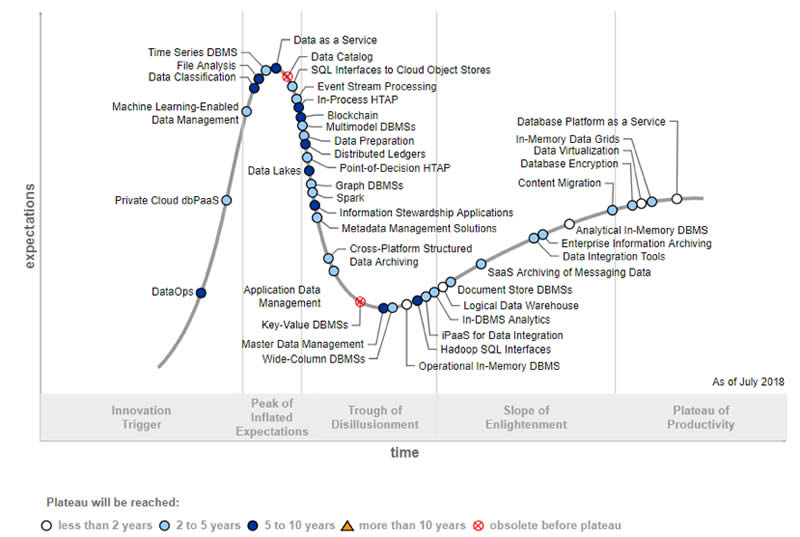
Data management FAQ
DAMA International defines data management as the development, execution, and supervision of plans, policies, programs, and practices that deliver, control, protect, and enhance the value of data and information assets throughout their lifecycles.
Data governance is one of the essential knowledge areas in data management and is closely related to data quality, another data management discipline. A data governance strategy consists of processes to maintain quality data throughout its lifecycle. As the foundation of data management, data governance i.a. defines how and why data will be used, the purposes for which data is managed/used, and how accuracy, security, and compliance are guaranteed.
Data management is the practice of managing data as a business asset and unlocking the value of data while ensuring it is accurate and protected. A good data strategy helps deliver the right insights and actionable information at the right time and place for the right purpose and data consumer (human or machine). Data management methods also enable data analytics. Data and data analytics are the enablers of digital business and the digital economy.
Top image: picture taken at CeBIT