Every organization must be using AI in some (more or less) mature way to make the most out of all that big data now, right? Not really. The market for machine learning-based intelligence is still in its infancy a survey says – and the challenges of data professionals are an important factor.
Most data professionals indicate that cleaning and organizing data is still the main challenge in their field. It indeed seems that the ‘good old’ 80/20 ratio of data science is still alive and kicking after many years.
Even in the ‘rather early days’ of big data it was estimated that data scientists (and other data professionals) spent anywhere from 50 to 80 percent of their time on data cleaning and ‘data wrangling’.
Individual contributors like data scientists and data analysts are more likely than managers and executives to list “connecting to data” and “deploying models into production” as challenges. This makes sense, as they are day-to-day struggles that directly impact their ability to be productive.
The estimate quickly became 80 percent in the communications of ample companies who had an interest in doing so and is one of those often-mentioned statistics we keep hearing since years. However, even in 2019, a full five years after the first time it was mentioned, reports and surveys still find similar results.
Cleaning dirty data – and structuring it – is essential of course. If it isn’t properly cleaned, labeled and so forth you can’t really rely on the output of what you do. To use an even older classic: GIGO – Garbage In, Garbage Out. Yet, it shouldn’t take too much time.
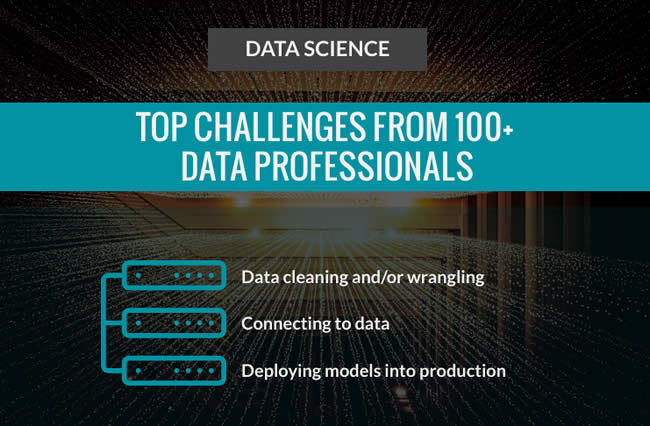
Data wrangling and connecting data sources: ongoing challenges for data professionals
We were reminded of the 80 percent statistic regarding the activities of data professionals when receiving a communication earlier in May 2019 from Dataiku.
The enterprise AI and machine learning platform supplier surveyed over 100 data professionals at its EGG Conference and found that around 80 percent of them still cite data cleaning and/or wrangling as their top challenge, followed by the challenge of connecting data.
Executives are starting to realize that transformation into a data-driven company doesn’t simply mean slapping data on top of existing processes.
Whether that means that 80 percent of the data scientist’s time is still spent on data preparation is – another – and undoubtedly individual – matter but the results are clear: ‘dirty data’ is still seen as a challenge and by all respondents, regardless of their functions (data scientists, data analysts, data team managers and other data professionals) it is mentioned as a daily struggle.
With the sources and volumes of data – mainly unstructured data – continuing to grow (IoT, for example, is only starting for most) that’s not the best news ever. Access to data sources is obviously also fundamental so it’s not positive that this is the second most mentioned challenge either.
For Dataiku the findings from its survey show that the market for machine learning-based intelligence is still in its infancy – as mentioned.
Fundamental challenges must be solved as data is paramount for AI and ML projects
For data professionals such as data scientists and analysts connecting data sources is seen more often as a challenge than by data team leaders. And the same goes for a third challenge, the deployment of data models into production.
Yet, this makes sense, as they are day-to-day struggles that directly impact their ability to be productive, Dataiku states. The results also mean that the main data problems are not about which model to use or even about how to make sure that the data team and stake holders collaborate, it’s still way more fundamental.
Hylke Visser, who is responsible for sales and business development for the Benelux region at Dataiku, recognizes that the findings aren’t surprising for data professionals since they only confirm what data scientists and analysts keep facing each day.
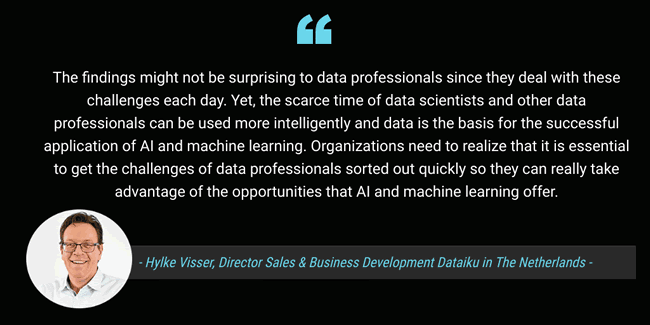
However, it’s important to continue to pay (even more) attention to it – precisely because it remains an issue, the scarce time of data scientists and other data professionals can be used more intelligently and data is the basis for the successful application of AI and machine learning.
Organizations need to realize that it is essential to get the challenges of data professionals sorted out so they can really take advantage of the opportunities that AI and machine learning offer, Visser adds. Obviously Dataiku also has a solution to lessen the challenges – through the use of automation with the development of AutoML having spurred the application of automation to the whole data-to-insights pipeline.
The responsibility for data and for data science (analytics)
Another – important – topic tackled in the survey is the question regarding responsibility for the organization’s data.
In these times of data protection, heightened awareness regarding privacy and from the AI viewpoint, ethical and responsible usage of data, it’s clear that data must not just be that proverbial new oil or gold and whatnot but also something to take really care of.
As topics like trust, bias, ethics, responsibility, transparency, and interpretability come to the forefront in machine learning and AI, the importance of a collective sense of responsibility for the company’s data itself might become more clear.
Only 16 percent of respondents thinks that data is everyone’s responsibility. This is worrying to some extent given the stricter rules regarding the protection and usage of data, among others with personal data protection in mind. Moreover, data is more often becoming a shared responsibility and topics such as trust, transparency and ethics are increasingly important when it boils down to machine learning and AI.
The importance of a shared sense of responsibility for the data of the organization becomes clearer due to these evolutions Dataiku states. If too few people are held responsible, however, this leads to incorrect use, errors and potentially irresponsible data practices.
Where it concerns the responsibility for data science (analytics) in organizations, most respondents indicated that everyone is responsible in a certain way.
Transforming into a data-driven organization
This is a positive sign for the future Dataiku says, emphasizing it means that people realize that to transform into a data-driven organization – quite essential in digital transformation – more is needed than just making data available for existing activities.
It means a fundamental organizational change where data must be interwoven in all processes of the organization Dataiku concludes. With the (coming) increase of data sources such as smart sensors and devices from the industrial applications of Industry 4.0 and IoT in mind it’s perhaps even more urgent to make that shift and make sure the data house is in order, time of data professionals is optimally spent, responsibility is taken care of and the increase of data gets properly leveraged for projects using AI, machine learning and thus valuable outcomes in an ethical way.
Dataiku bundled the takeaways from the survey with a split per industry, thoughts on enterprise AI and additional recommendations in a white paper, entitled ‘From the trenches: a survey report – insights on top challenges from 100+ data professionals’.
The EGG Conference series of Dataiku (‘The human-centered AI conference’) is also about to start again with a first stop in New York in June, followed by London (July), Paris and San Francisco.
These days it’s very hard not to be convinced that AI is omnipresent but we’re not quite there. It remains key to, as IBM’s Ginni Rometty put it at the VivaTech 2019 event in Paris, ‘get the data in shape’ – and that is still a challenge.
As said, it isn’t new. Yet, as the survey from Dataiku indicates it still plays.
Dataiku is an originally French startup, founded in 2013 to solve a challenge in data science: a better collaboration between data scientists, data engineers and business analysts. The company is now active across the globe (also in Australia since early 2019) and in 2019 again was named a challenger in Gartner’s Magic Quadrant for Data Science and Machine-Learning Platforms – for the third year in a row.
All pictures courtesy of their respective mentioned owners.